Competitive data in the short-term rental market gives managers answers to important questions regarding their pricing strategy. This type of data is also used in revenue management and pricing algorithms to optimize price. For data to be predictive, it needs to be both accurate and representative. Selecting the correct data that checks both of these boxes can be a challenge.
Types of Data
Scraped Data
The term scraped comes from the term screen scrape. Initially, these would be programs that would visit websites and extract the data from the screen. Although some methods have improved, the principle of scraping data off the internet remains the same. Data is collected by visiting a company’s website and extracting the information of your interest. Before developers wrote programs to collect this data on a large scale, analysts and managers would collect data by browsing websites manually. This process is highly time-consuming, depending on how much information you want to capture. Many owners and property managers still browse these sites manually to verify prices. In our industry, managers are very interested in what their nearby competitors are charging, their occupancy rate, and how they stack up. Online Travel Agencies (OTA) data scraping is a reliable way to collect competitors’ data in bulk and start answering questions. The vast majority of the data used in the short-term rental market is collected this way.
Anonymized Host Data
Scrape data comes from accessing a frontend of an application or website. In contrast, Host data comes from the manager, owner, or host of the property. As a property manager or owner, you can access this data for all of your rental units. But your data doesn’t give you the insights into your competitors that you are likely after. In the hospitality industry, several providers sell host data to the marketplace. This data is from other hosts in your area. The arrangement with the buyers of this data is that they are also suppliers. As part of the agreement to access other properties’ host data, they must also contribute data into the pool. Then the data is aggregated, which maintains the anonymity of the other hosts.
Data Accuracy and Limitations
The purpose of competitive data is to help managers better understand their market and their competitors. This better understanding allows them to make better choices regarding their pricing and minimum night stay strategy, which leads to better unit revenue.
Scraped Data
Scraped data has its uses as a data source, but it is simply the least reliable and least accurate type of data available. That is not particularly surprising when you think about how it is collected. A much easier way to get the same data would be to ask Airbnb or VRBO for keys to their APIs. That would eliminate the need for repeatedly pinging their front-end for information.
Many reasons are in place for this to be improbable, so the only way to get the data is to create it by making tons of requests to their website and interpreting the responses. In dynamic markets, prices change frequently. Professionally managed properties can have the prices change several times in a single day. That is a substantial challenge for data scraping because running the scraper at a high frequency and storing that much data becomes very expensive very fast. When the scraper’s frequency is not enough, it will miss the critical changes.
Just to give you an idea of scale, handling data for a single unit’s daily pricing for the next 365 days throughout an entire year could take up to approximately 20 MB of data, granted this update performs only once every day. That sets up the average block of data at 1GB for every 50 tracked units year-round. Now keep in mind that an average city can have anywhere from 2,000 to 5,000 units, and metropolitan areas can have anywhere from 20,000 to 30,000+ units in their market. Also, consider new properties, properties re-listed, and properties dropping off the market.
Occupancy can also be challenging to calculate from scraped data accurately. Scrapers see a binary value for occupancy “occupied” or “not occupied,” but there is a third value that they cannot see. That value is “owner-occupied.” There are also segments of the inventory that the manager may block off for multiple reasons. Inventory could appear as booked to the scraper, but the manager is just holding it off the market for some time.
Similarly, some properties will show as booked or canceled. The combination of pricing and occupancy having accuracy issues makes calculating revenue challenging to get correct. Quibble ran a data scraper on a property set for which we also had the host data. The experiment ran over six months on 100 properties across the US. Below are the results:
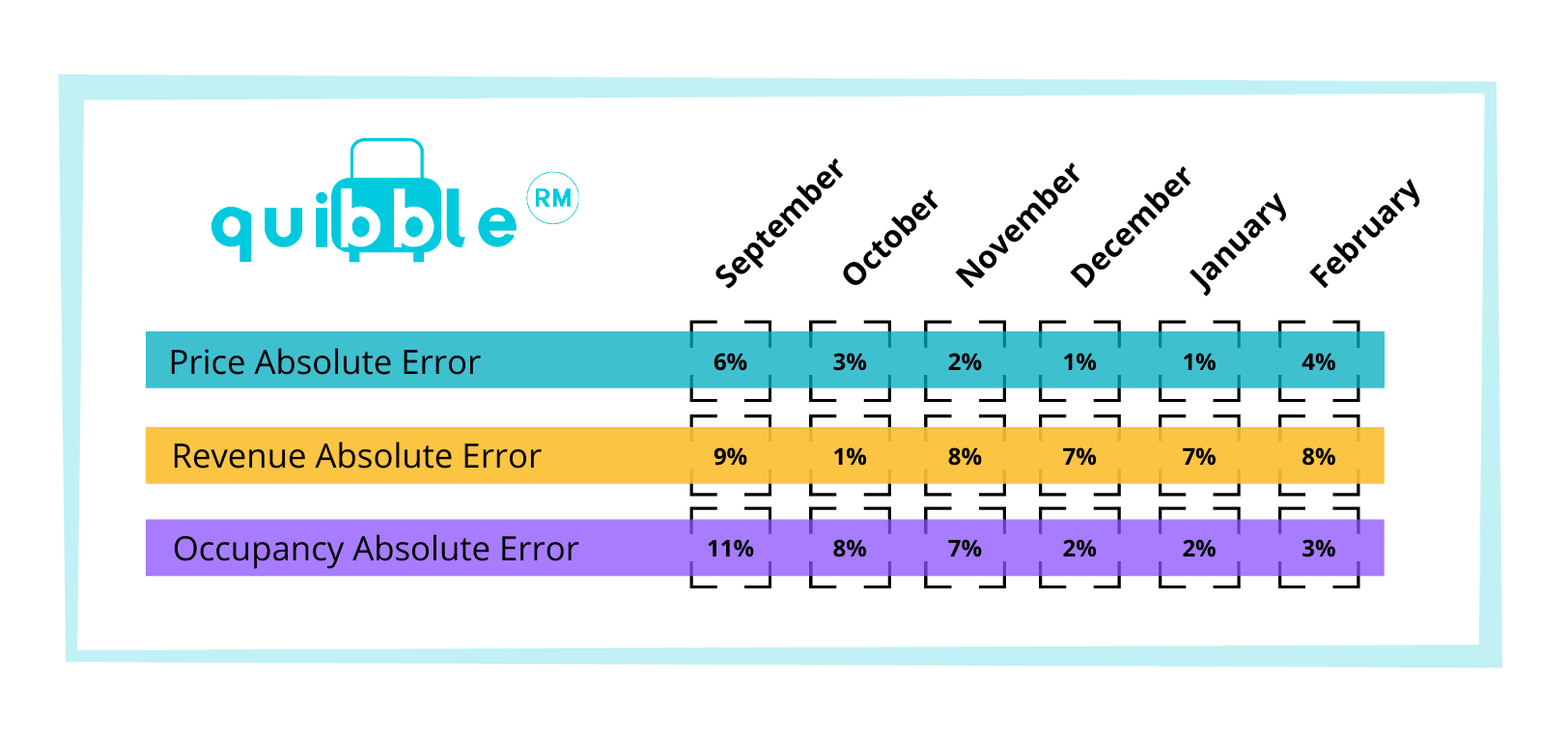
The experiment’s purpose was to test the data’s accuracy and determine its value for incorporating it into our pricing algorithm. The error is the difference between what was captured by the scraper and the actual host data. How meaningful is this error? That depends on the purpose and intent you have for that data.
Anonymized Host Data
Host data is very reliable because the source is the host. The data does not have issues with pricing, occupancy, or revenue. The host sells every reservation, so they know the exact price for every reservation sold. Therefore they know the revenue. They also know the occupancy because they are controlling the inventory availability.
The most significant constraint to host data is availability. There are not many vendors offering this data to the STR industry at the moment. The most notable provider is Key Data Dashboard. To get this data, the vendor needs to create a relationship with many property managers in a single area. If a particular area only has 2-3 property managers or very few properties, the anonymized host data will not be beneficial. That also breaks down anonymity. But, once the market has enough participants (customers), the data becomes incredibly useful and reliable.
How valuable is this data? That depends on how much market penetration the vendor has in your market.
When and how to use each source of competitive data in the short-term rental industry
One of the most significant benefits of using scraped data is how quickly it’s generated without needing hundreds or thousands of reciprocal agreements. So, data scrapers and vendors that sell this data can get data on hundreds of thousands of properties very quickly. What the data lacks in accuracy, it makes up in quantity. On the other hand, the dataset from the anonymized host data is much smaller but is much more accurate.
The table below describes the appropriate use of each of the available data types.
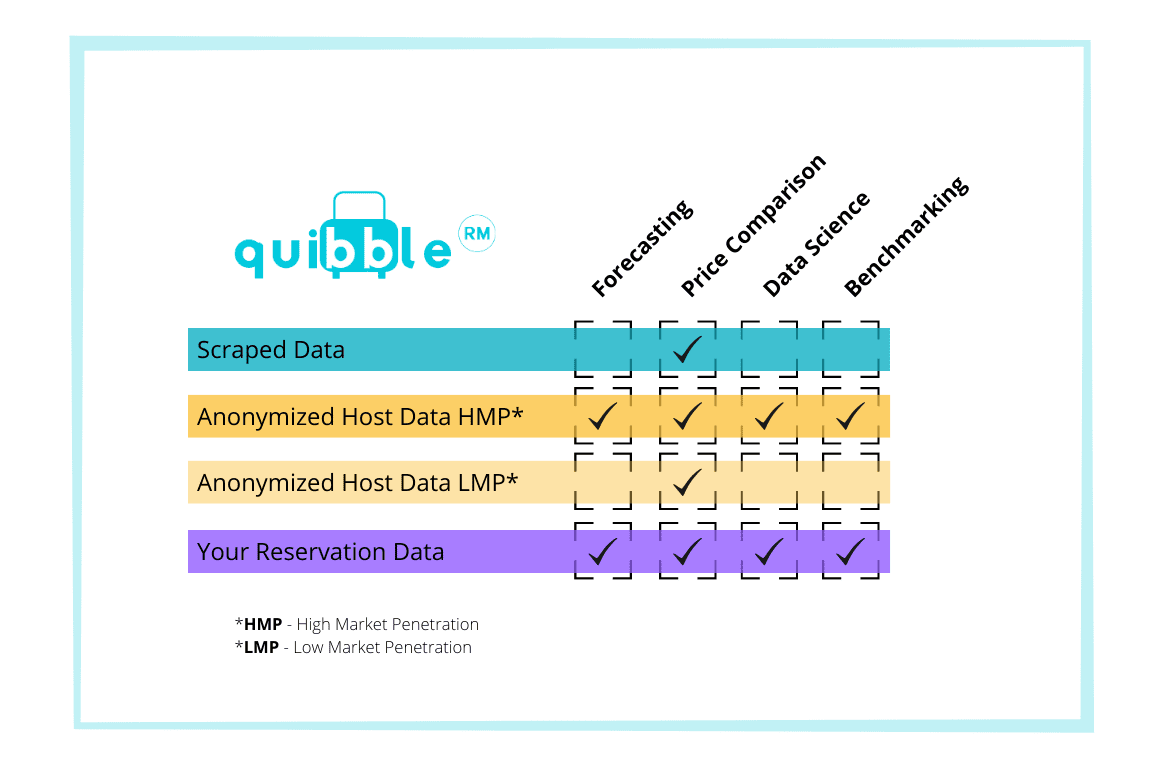
Since scraped data has the most significant error, it has the fewest functions in your toolkit. A good data scraper that is frequently running should have the lowest error on capturing competitor pricing. That makes this data great for price comparison.
All pricing and revenue management functions in your business can use anonymized data in a market with high penetration (+10% of the market represented). If the data has low market penetration, you should treat the data more like the scraped data.
A data source you should be 100% confident in is your reservation data. This data is excellent for all functions of your business.
Conclusion
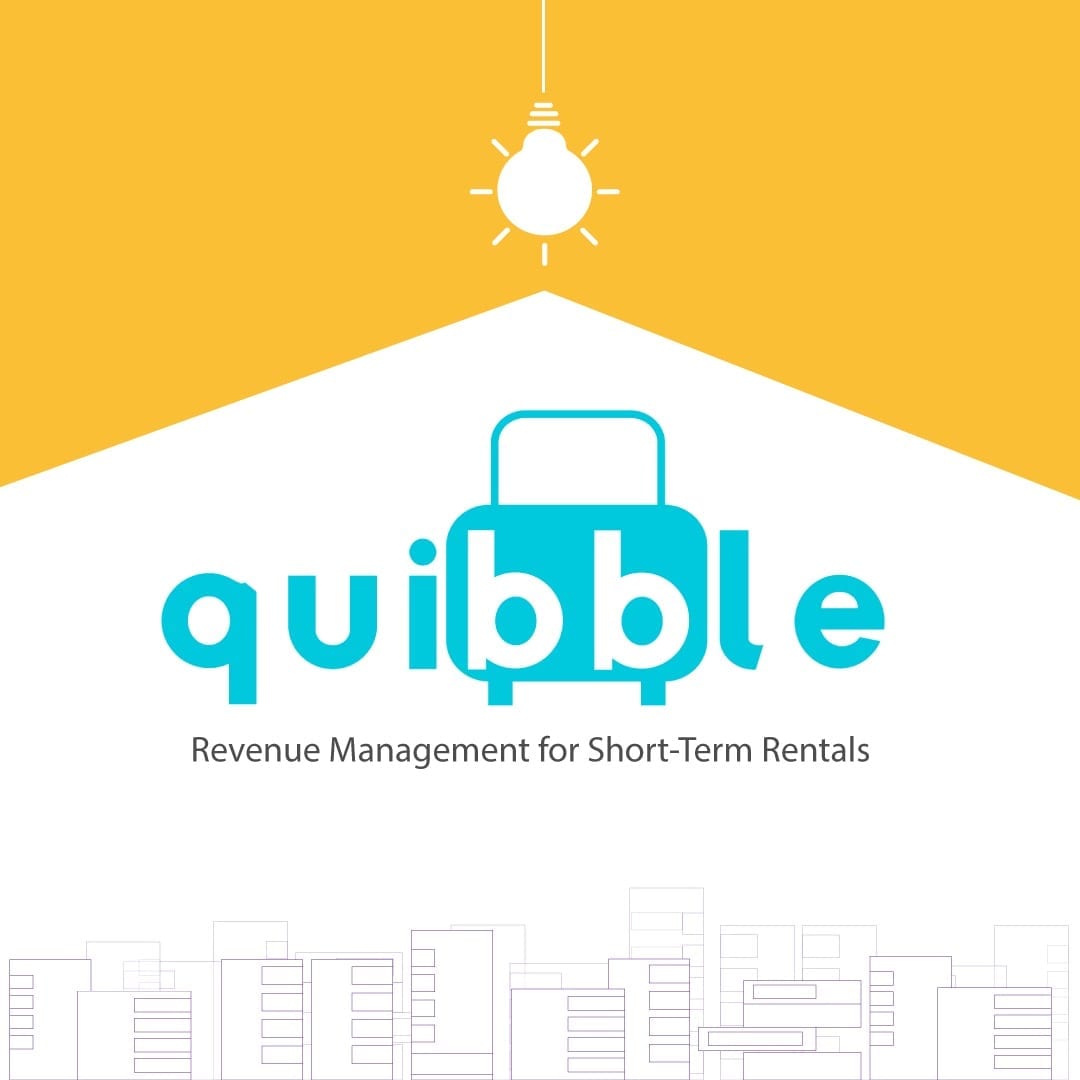
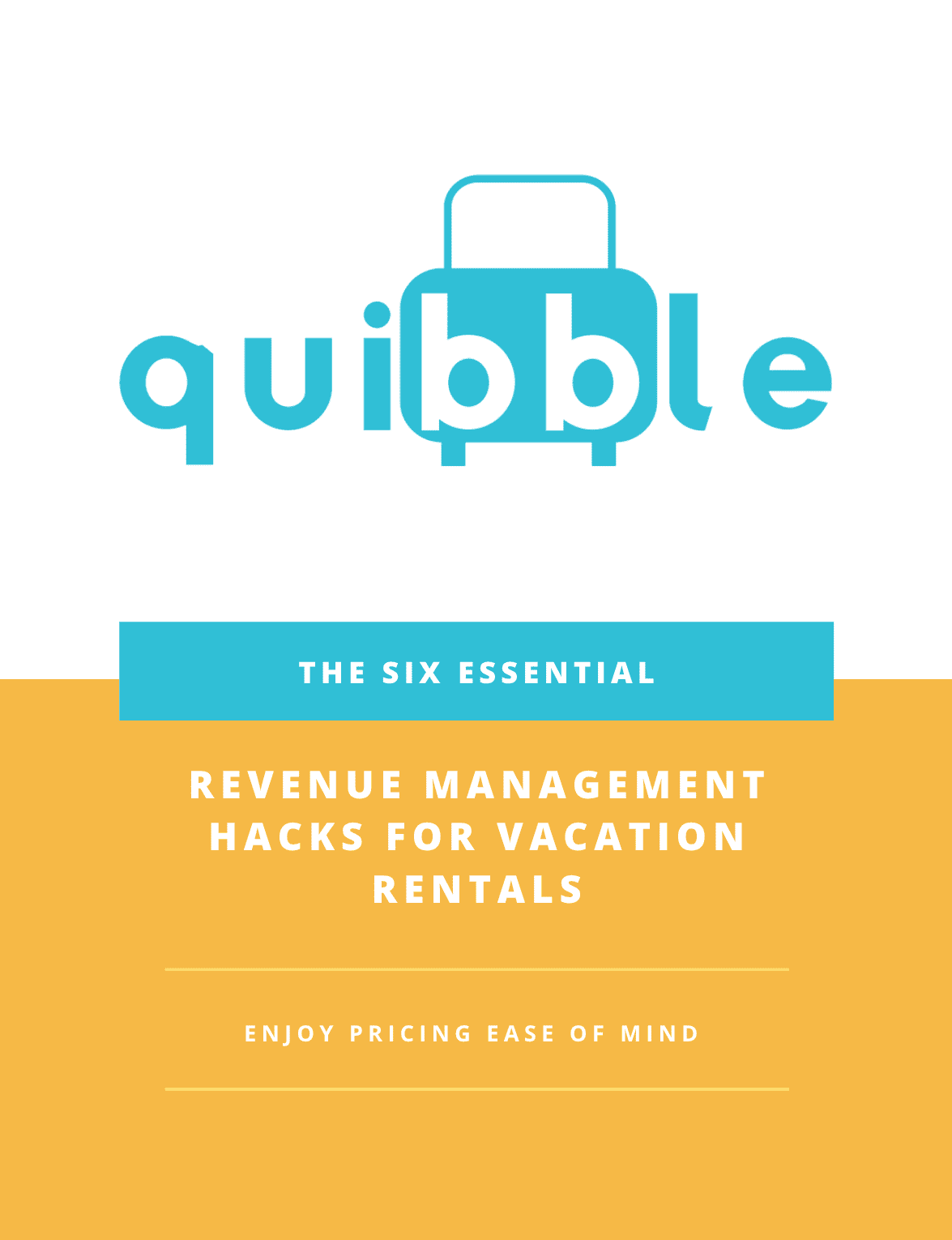
Join our newsletter
Dominate the short-term rental market with cutting-edge trends