Revenue Management
The process of adjusting prices to better match demand is called revenue management and is a form of price discrimination. It is a practice widely used today to increase revenues in many industries. A base price is a concept inside the practice of revenue management, specifically hotel revenue management. In the days before revenue management or dynamic pricing, the price for a hotel room night was a single rate. It didn’t matter what day it was or what season it might have been. Even though the managers were aware of the seasonal patterns of demand, managing a variety of price points for various room types was prohibitive because they lacked the required technology.
“Even though the managers were aware of the seasonal patterns of demand, managing a variety of price points for various room types was prohibitive because they lacked the required technology. ”
The technology required to control and distribute prices efficiently didn’t exist until the 1980’s when computer reservation systems became connected. Automated pricing software began to integrate into these new reservation systems, opening the possibility of managing more price points efficiently. Pricing at a very granular level became technologically feasible to manage and distribute multiple price points. The marginal gains from improved pricing were substantial and encouraged the development of pricing and revenue management science to enhance pricing and forecasting algorithms.
Base Price
Base price is a method to implement a revenue management strategy and increase pricing variability. The base price method is one of the simplest and easiest revenue management methods to understand and implement. Imagine the hotel that managed their pricing with just a single price. That price was very likely a little too high for the off-peak season and a little too low for the peak season. The hotel manager might want to increase pricing in the peak season by 30% and decrease it in the low season by 25%. So, they take the starting price, which becomes the base for their adjustments, and add 30% or subtract 25% depending on the season. The base rate is the starting point for all price adjustments.
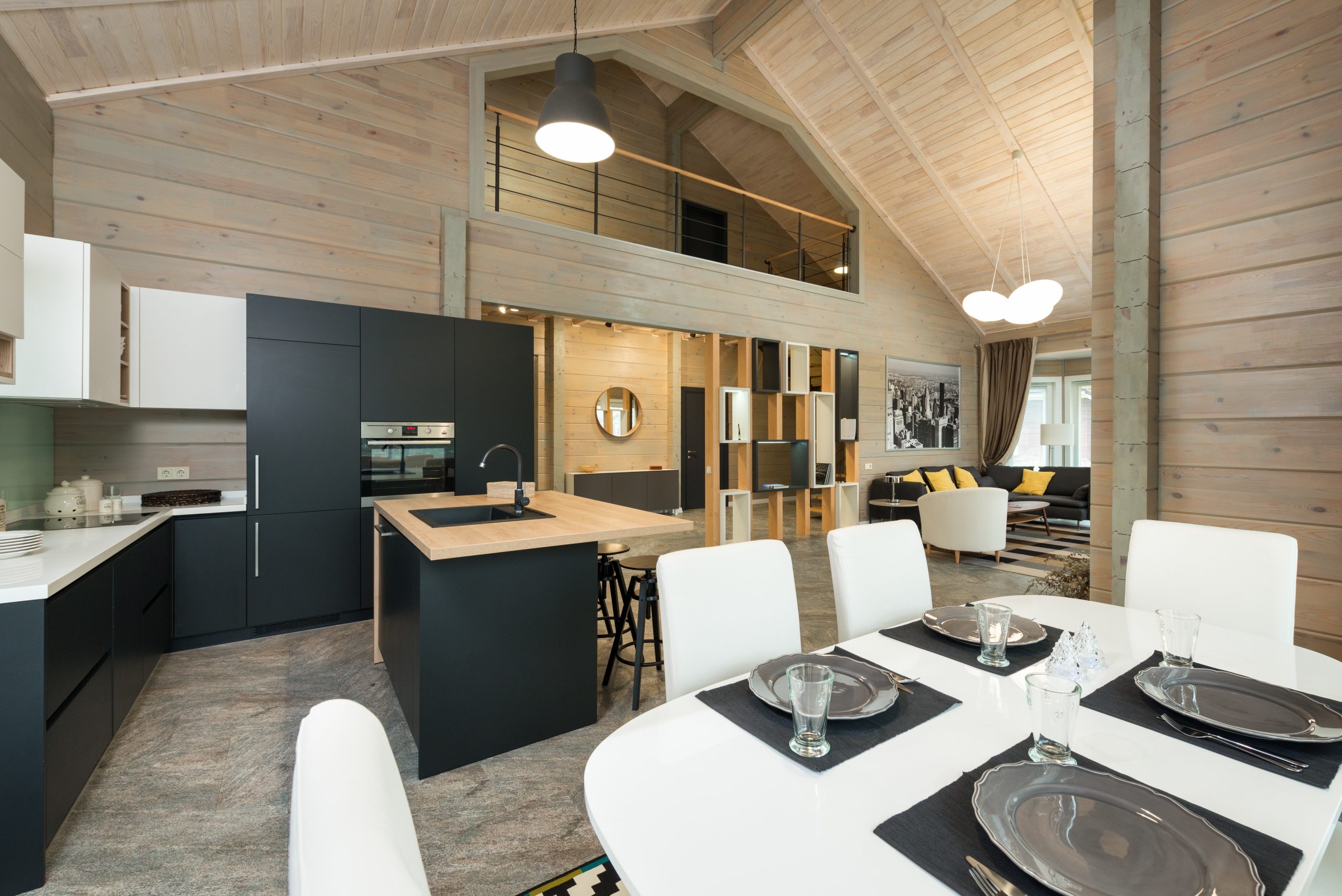
Revenue management strategies can increase in complexity from there. For example, demand for Mondays can differ from demand for Fridays, and the adjustments to the base price can reflect these differences. In addition, much of the pricing strategy used in the hotels is through different channels, which can increase complexity exponentially. Each distribution channel can have its pricing strategy, which is all applied to the base price.
Use in Short-Term Rentals (STR)
Current pricing methods in short-term rentals (STR) have adopted the base price methodology from the hotels. When starting a new industry, it is easy to use what is already working somewhere else, and the similarity with hotels was close enough. The STR industry uses a form of dynamic pricing that makes adjustments based on what their neighbors in the surrounding area do. The current applications take their neighbor’s price and watch for changes to their price. When the applications see a change in the neighbor’s price, they assume that the neighbor has discovered a change in demand in the market. If the neighbor drops their price by 9%, the application drops your price by the same. The adjustments are determined and applied to the base price the user has set.
“The current applications take their neighbor’s price and watch for changes to their price.”
The data below shows an example of how to calculate the multiplier. First, the application needs to know what the neighbor was charging yesterday. Next, the application checks what the neighbor is charging today to see if the price has changed. Then calculate the percentage change in price and apply that to the Base Price. Every time your neighbor changes its price, the application updates the Base Price multiplier.
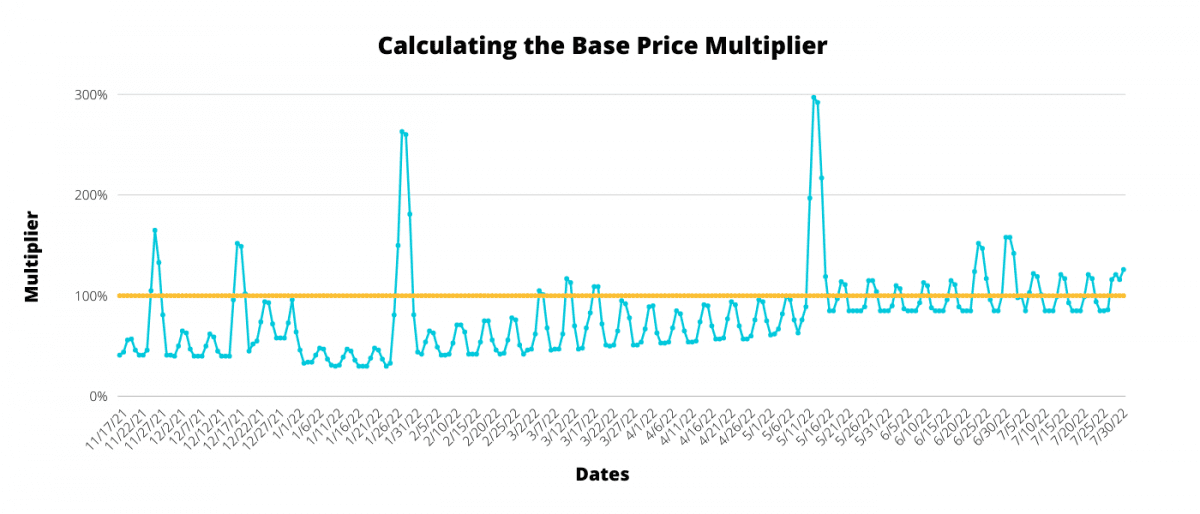
The neighbor model is a novel solution to dynamic pricing and a different approach to adjusting base prices than its hotels and airlines’ predecessors. But in the early days of STR dynamic pricing, the data was limited, and running robust forecasting models was impossible. The revenue management models used by hotels and airlines rely heavily on reservation data, which is historical by nature. Many STR managers didn’t have enough data to power these more scientific forecasting models. As a result, the neighbor model is still the dominant method of STR dynamic pricing.
Base Price Method Problem
The first assumption of the base price model is that the user knows what the base price should be. It is the foundation for all multipliers and adjustments, so getting it right is critical to optimizing daily rates. Dynamic pricing applications will often recommend a base price at the property level, and they can use two different methodologies to do this. They can either look at other properties in the area using data scraped off of an OTA or historical reservation data for a property.
The first method, Scraped Data, can be a logical starting point for a brand-new property with no historical reservation data since the available information is limited. However, this method doesn’t perform well when pricing a unique property that doesn’t compare well to the surrounding market. It also depends on your neighbor having priced their properties appropriately because they are your benchmark. If your neighboring property pricing is inadequate, your property’s price will also follow the same inadequacy.
“If your neighboring property pricing is inadequate, your property’s price will also follow the same inadequacy.”
The second method, which involves using the property’s historical reservations, is more reliable because it doesn’t have this dependency. However, it runs into problems when the property is not priced correctly historically.
These methods can work under specific conditions but leave plenty of room for error. As a result, frequently base prices are set with neither of these methods. Instead, property managers rely on assumptions and hopes regarding what the property can demand in the market.
Next comes the maintenance of the base price itself. After setting a base price, the strategy requires the user to make any future adjustments to the base price and maintain it. It is a singular stored value in the system. An additional complexity driven by the STR market is the high volatility of both supply and demand. For that reason, it is unlikely this year’s base price will be the revenue-maximizing price for next year, or possibly even next month. That puts a demand on the revenue manager to continuously update the base prices for all the properties under their management or risk that they are no longer optimal for the new market conditions. This very tedious task for managers gets easily overlooked when managing large portfolios of properties.
“It is unlikely this year’s base price will be the revenue-maximizing price for next year, or possibly even next month.”
Seasonality offers another challenge to this methodology. Some markets have extreme differences in demand for the high season as it does for the low season. For example, summer lake houses and ski cabins can see four times higher demand in their peak season compared to the off-season. It is challenging for a base-priced methodology to find and apply a 400% increase to the season because it relies on your neighbors to make that exact 400% change. Managers in these seasonal markets are the most resistant to using dynamic pricing. This reason primarily drives that decision; they can’t account for these significant differences between seasons. Taking just one booking at a low price during peak season is enough to cause a manager to swear off dynamic pricing altogether.
Solution
The next generation of STR revenue management, QuibbleRM, has replaced the Base Price with an Optimized Price derived from a regression model. The model takes all the relevant reservation data and creates a revenue-maximizing price automatically. Every new reservation gets automatically added to the model, and the optimized price updates continuously. These updates are made in real-time and are generally minor price adjustments. But, the system will always be moving the price to meet supply and demand and maximize the revenue over a more extended period.
“The system will always be moving the price to meet supply and demand and maximize the revenue over a more extended period.”
Originally the Base Price concept came to the short-term rental industry through its strategic proximity to hotels. But as anyone in this industry can tell you, it’s surprisingly different in pricing than vacation homes. Today, many property management companies have enough data to power a robust pricing model, which wasn’t true when the original industry models got incorporated to price STR’s dynamically. Revenue managers no longer need to set base prices, maintain base prices, or worry about extreme seasonality. These are all problems that can be left to a machine to handle.
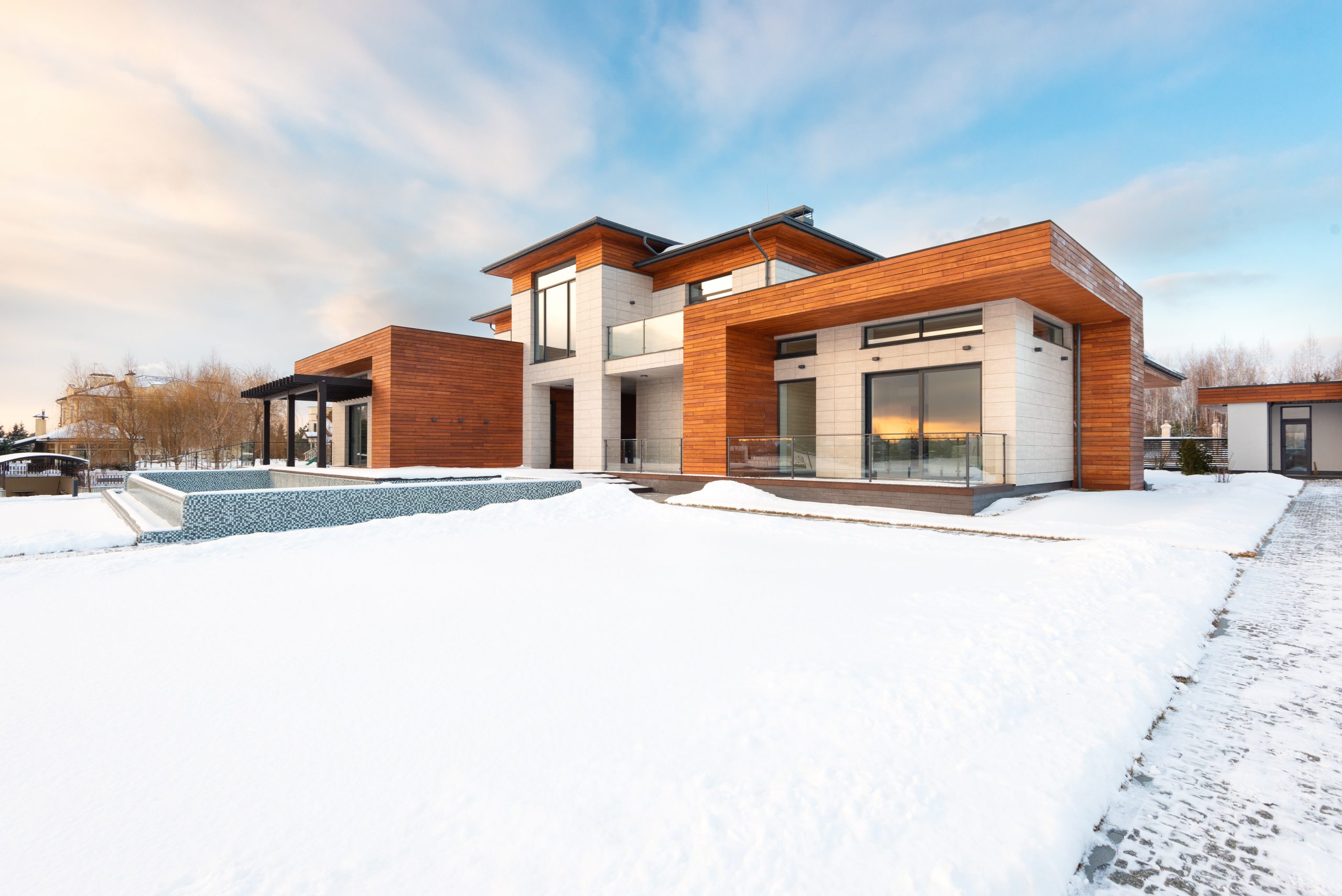
QuibbleRM has an embedded forecast feature that automatically segments demand into Season/Weekday history pools. The optimized prices are unique to each day of the week and season. Base price models rely on a single base price for each property. The single base price limits how much of the weekday demand and seasonality pattern can be captured by this model. Quibble segments demand on a much more granular level. The system generates a minimum of 21 optimization prices, allowing more potential revenue capture. That enables the system to quickly find microtrends that develop on certain days of the week or season. The concept of 21 prices per property to manage was previously unmanageable because the user was manually creating and managing these prices. Using this pricing model opens the possibilities for increased revenue capture.
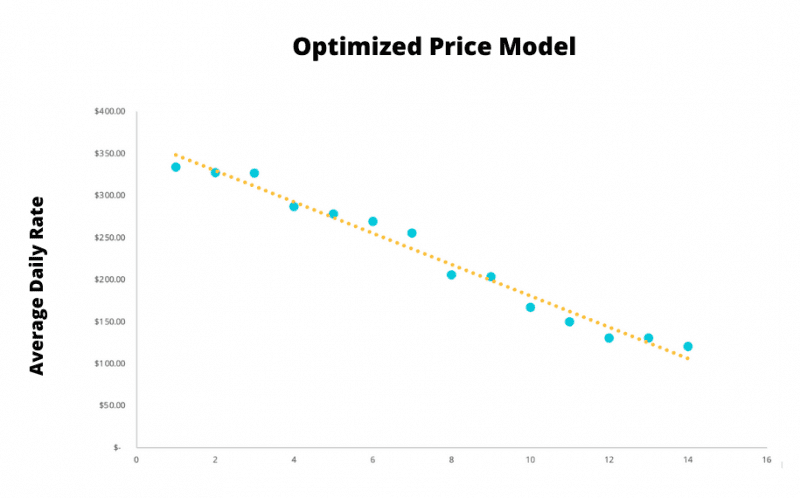
Quibble’s optimal price method ensures that the pricing improves over time. Regression modeling is a form of machine learning that helps predict future events. They allow the system to learn if prices are trending up or down and then react to those changes without requiring the revenue manager to engage in the process manually. We test 6 different models for fitting accuracy every time the model runs, which improves our ability to match supply with demand as they shift quickly.
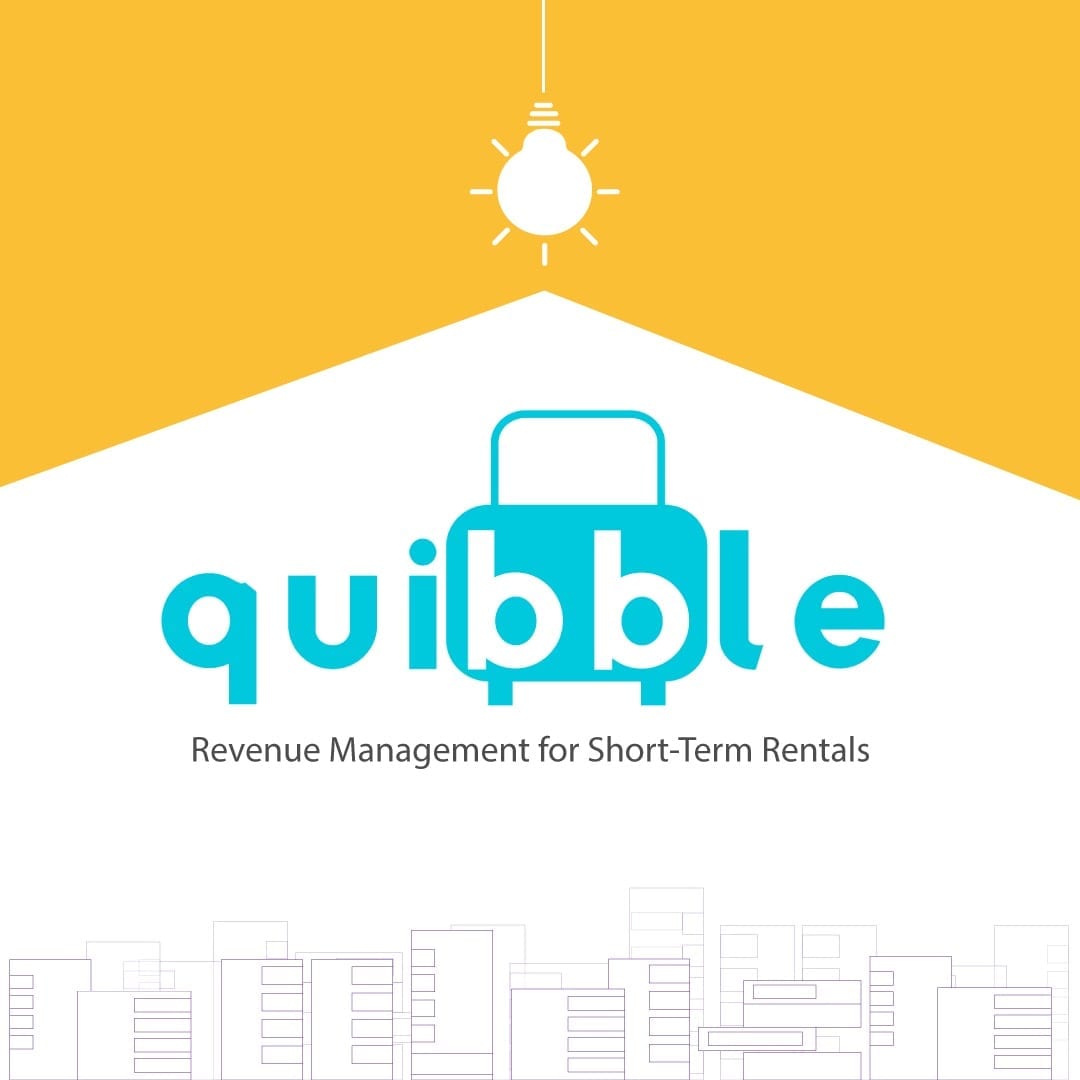
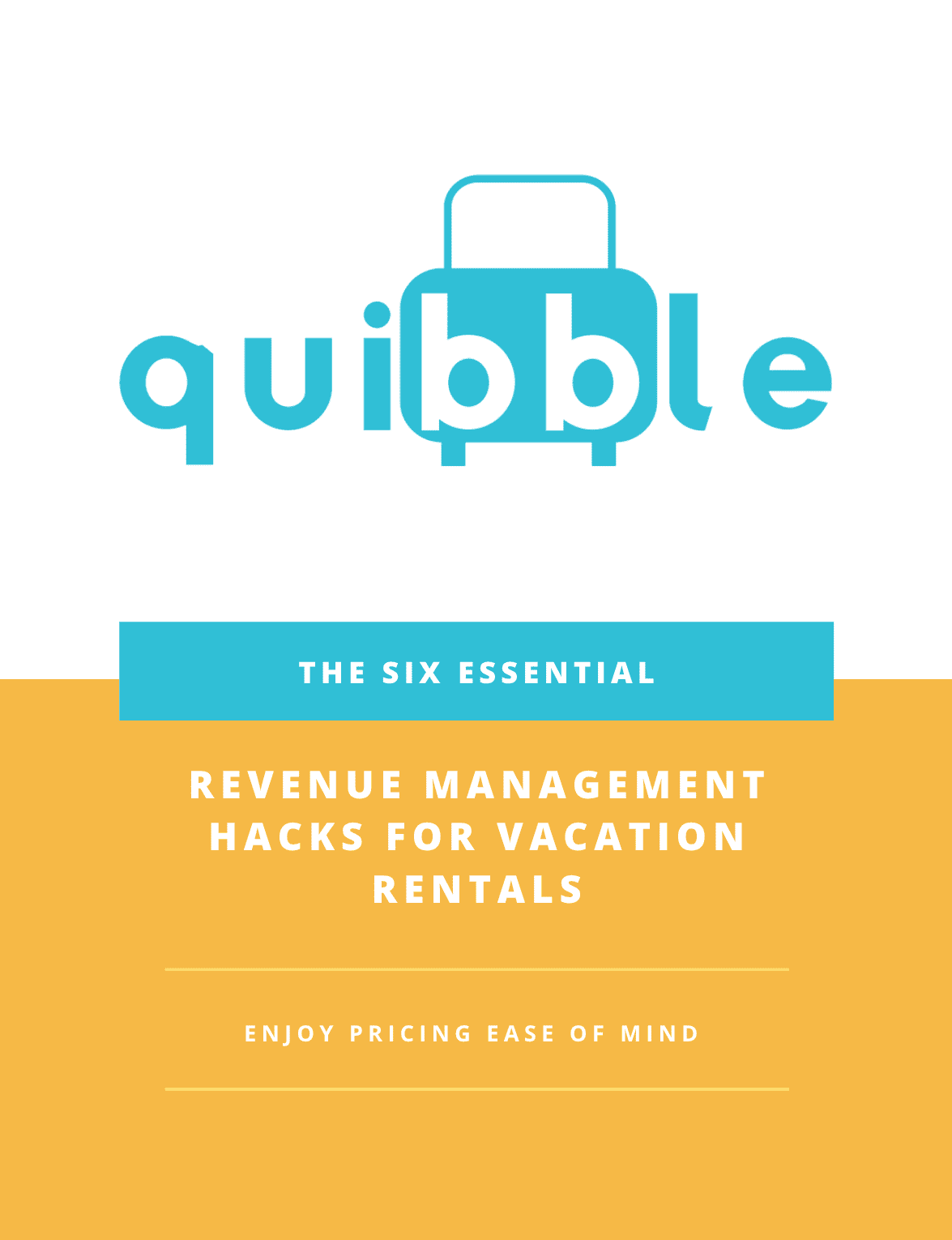
Join our newsletter
Dominate the short-term rental market with cutting-edge trends